My colleagues and I instead hope to build the first dedicated neural network computer, using the latest “quantum” technology rather than AI software. By combining these two branches of computing, we hope to produce a breakthrough which leads to AI that operates at unprecedented speed, automatically making very complex decisions in a very short time.
We need much more advanced AI if we want it to help us create things like truly autonomous self-driving cars and systems for accurately managing the traffic flow of an entire city in real-time. Many attempts to build this kind of software involve writing code that mimics the way neurons in the human brain work and combining many of these artificial neurons into a network. Each neuron mimics a decision-making process by taking a number of input signals and processing them to give an output corresponding to either “yes” or “no”.
Each input is weighted according to how important it is to the decision. For example, for AI that could tell you which restaurant you would most enjoy going to, the quality of the food may be more important than the location of the table that’s available, so would be given more weight in the decision-making process.
These weights are adjusted in test runs to improve the performance of the network, effectively training the system to work better. This was how Google’s AlphaGo software learned the complex strategy game Go, playing against a copy of itself until it was ready to beat the human world champion by four games to one. But the performance of the AI software strongly depends on how much input data it can be trained on (in the case of AlphaGo, it was how often it played against itself).
Our Quromorphic project aims to radically speed up this process and boost the amount of input data that can be processed by building neural networks that work on the principles of quantum mechanics. These networks will not be coded in software, but directly built in hardware made of superconducting electrical circuits. We expect that this will make it easier to scale them up without errors.
Traditional computers store data in units known as bits, which can take one of two states, either 0 or 1. Quantum computers store data in “qubits”, which can take on many different states. Every extra qubit added to the system doubles its computing power. This means that quantum computers can process huge amounts of data in parallel (at the same time).
So far, only small quantum computers that demonstrate parts of the technology have been successfully built. Motivated by the prospect of significantly greater processing power, many universities, tech giants and start-up companies are now working on designs. But none have yet reached a stage where they can outperform existing (non-quantum) computers.
This is because quantum computers need to be very well isolated from disturbances in their surroundings, which becomes harder and harder as the machines get bigger. For example, quantum processors need to be kept in a vacuum at a very cold temperature (close to absolute zero) otherwise they could be affected by air molecules striking them. But the processor also needs to be connected to the outside world somehow in order to communicate.
More room for error
The technical challenges in our project are very similar to those for building a universal quantum computer that can be used for any application. But we hope that AI applications can tolerate more errors than conventional computing and so the machine won’t need to be quite so well isolated.For example, AI is often used to classify data, such as deciding whether a picture shows a car or a bicycle. It doesn’t need to fully capture every detail of the object to make that decision. So while AI needs high computer speeds it doesn’t demand such high levels of precision. For this reason, we hope that makes AI an ideal field for near-term quantum computing.
Our project will involve demonstrating the principles involved with a quantum neural network. To put the technology to its full use will involve creating larger devices, a process that may take ten years or more as many technical details need to be very precisely controlled to avoid computational errors. But once we have shown that quantum neural networks can be more powerful than classical AI software in a real world application, it would very quickly become some of the most important technology out there.
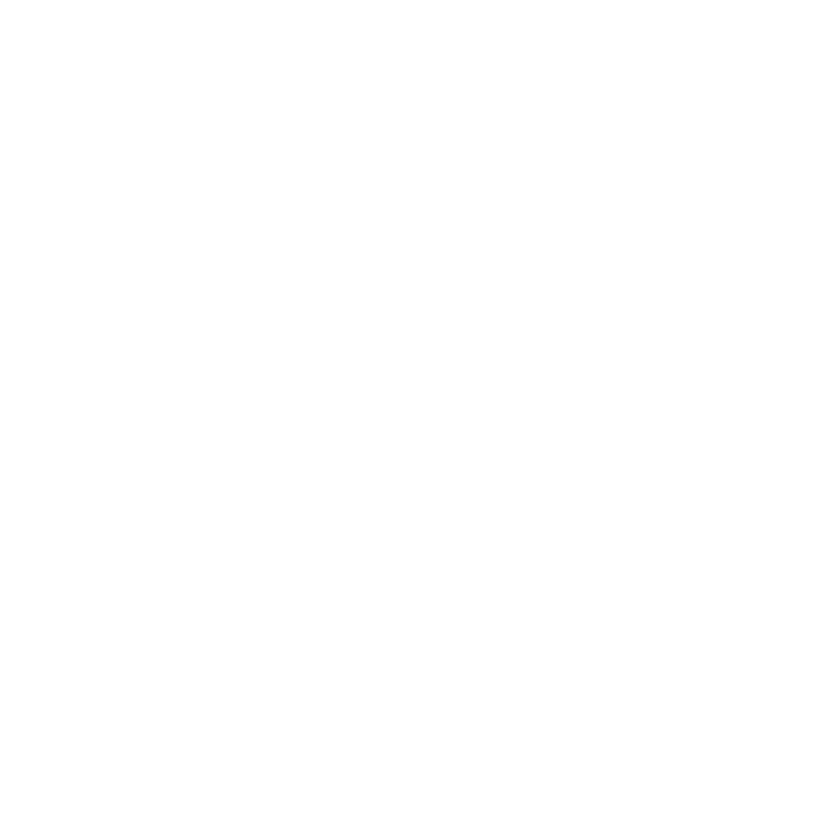
This article is republished from The Conversation under a Creative Commons license. Read the original article.