Introduction
From remediating blight to optimizing restaurant inspections and pest control, cities across the country are using analytics to help improve municipal policy and performance. The continued adoption of analytics in city governments shows no sign of slowing, and as even more sophisticated tools such as machine learning and artificial intelligence are deployed, there is a critical need for research on how these practices are reshaping urban policy. By examining and capturing lessons learned from city-level analytics projects, practitioners and theorists alike can better understand how data- and tech-enabled innovations are affecting municipal governance. This report seeks to contribute to that developing field.
A key component in creating, launching, and implementing an analytics project is adopting a systemic approach to project development. Whether a city has an established chief data officer position, an analytics team, or is a newcomer to public-sector data analytics altogether, there are various approaches and processes that can help initiate, scope, and implement a successful analytics project.
While many urban analytics projects have been largely successful in fulfilling their initial objectives and supporting better governance, most underwent multiple iterations. Furthermore, the continued expansion of data use in city government raises complex ethical questions that go to the core of American democracy. While more and more sophisticated technologies are being deployed to help improve government’s ability to serve the public, those new tools may be operating on assumptions or biases that will exacerbate, not ameliorate the challenges facing our most vulnerable populations. To successfully navigate this dynamic and rapidly evolving intersection of public policy and technology, government, at all levels, desperately needs better information on the state and implications of these new practices to help practitioners and academics alike find sustainable, equitable solutions.
an·a·lyt·ics / anəˈlidiks/ noun - models of data analysis that provide predictive or forecasted insights via statistical analysis.
How to Approach Analytics Project Development
If a department, agency, or city is considering using analytics, there are a few ways to get started. While some organizations follow general standards of practice to provide a step-by-step guide on the key phases of project development, others follow a technical framework to identify the degree to which organizational and/or data resources will support a given project. Drawing on the various approaches, process guides, and methodologies developed by Civic Analytics Network cities and partners, this report highlights five key steps that cities can replicate to develop their own analytics projects: (1) identify the problem; (2) assess data readiness; (3) scope the project; (4) pilot the project; and (5) implement and scale the model.The first step the Civic Analytics Network recommends—before considering what data an organization has available—is establishing a clear understanding of the problem to be addressed by a given analytics project. Determining data readiness or maturity is critical, but before an analytics project can even be scoped, it is important to ensure that the project’s objective is core to the performance or needs of the implementing organization; data-driven policymaking is not data use for the sake of data use. After working with departments to identify a mission-critical problem, analytics experts or data scientists can proceed with identifying data readiness, scoping and piloting the project, and so on.
From forecasting future needs to overcoming staffing or resource shortages, to condensing vast and disparate information into actionable insights, analytics can be a powerful tool in improving city governance, but it is most effective when used to enhance and support the efforts and priorities of city personnel. There is no better way to ensure that than by positioning data scientists within government to work with departments on tackling key issues collaboratively. It is important to note that many analytics teams are small, nascent offices with restrictive funding resources for data experts to introduce the value of data analytics to their cities’ bureaucracy; many new analytics teams or hires are established thanks to the support of the bully pulpit, and the mayor can play an important role in prioritizing data use to address policy needs in local communities.
1) Identify the Problem
Identifying a critical problem that can be supported or alleviated by analytics is challenging, but it is an important first step in structuring a successful analytics project. While data may abound, matching an area of need with the right data resources within an organization is vital. Developing an analytics project typically places data scientists in an internal consultant role; by working with a department or agency to identify their key issues or problems, data scientists can support mission-critical needs. For example, the City of Chicago’s Applied Analytics Guide prioritizes working in partnership with departments and agencies across government as part of its ten-step process to develop advanced predictive analytics projects. Chicago was an early leader in city-level data-driven policy as one of the first cities to create a chief data officer (CDO) position, which is housed within the city’s Department of Innovation and Technology (DoIT).In Chicago, direct engagement with departments across city government helped DoIT build relationships that created an environment in which department leaders see the DoIT team as a resource to help them explore new solutions to key problems. DoIT does not prescribe data solutions, but rather supports the priorities of department leaders to optimize performance and/or service delivery in areas that the leaders identify as areas of need. Sometimes, however, public exposure of an underperforming service area can spur an analytics “intervention.” For example, following media coverage on restaurant inspections, DoIT helped craft an analytics model to help optimize restaurant inspections (see “Public Health” below).
Other cities similarly prioritize this problem identification phase as a way of developing meaningful partnerships with government agencies. Many Civic Analytics Network analytics teams are situated in their respective city governments as discrete groups tasked with supporting and collaborating with other departments or agencies by bringing data-driven insights and expertise to bear on key issues or areas of need.
- See “CAN Webinar: Predictive Analytics: A Ten-Step Guide” (Ash Center) https://datasmart.ash.harvard.edu/news/article/webinar-recording-predictive-analytics-a-ten-step-guide-1220.
- See “Mayor’s Office of Data Analytics (MODA) Project Process” (New York City) http://www1.nyc.gov/assets/analytics/downloads/pdf/MODA-project-process.pdf.
- See “A Starter Kit for Data-Smart Cities” (Ash Center) https://datasmart.ash.harvard.edu/news/article/a-starter-kit-for-data-smart-cities-1004.
- See “Case Study: Boston’s Citywide Analytics Team” (Ash Center) https://datasmart.ash.harvard.edu/news/article/case-study-bostons-citywide-analytics-team-1043.
2) Assess Data Readiness
Determining data readiness is a key facet of Civic Analytics Network cities’ approaches to analytics and a critical precondition to scoping any project. The success of an analytics project depends not only upon whether there is a need for data analytics, but also, and more importantly, on having the right personnel, data collection and storage practices, and stakeholder buy-in within and outside of the department or agency.The University of Chicago’s Center for Data Science and Public Policy (DSaPP), a Civic Analytics Network partner, created a “Data Maturity Framework” to help prepare prospective project leaders for the development process. The framework provides an effective structure to help determine data readiness for an organization considering a new analytics project. Drawing on its team’s experience sourcing project proposals, DSaPP observed a trend: most prospective project managers referred to a desire to make use of tons of unused data. While having ready-made or already assembled data is a good start for any analytics project, DSaPP needed to help project managers understand that successful analytics projects begin with the identification not of unused data, but of critical issues in need of data-driven solutions. Once that issue area or policy need was identified, then data scientists could help project managers assess data readiness and begin structuring and scoping an analytics model.
DSaPP’s Data Maturity Framework consists of a questionnaire and scorecards to identify the technology, data, and organizational readiness within a department. The framework consists of a questionnaire and survey to assess readiness and three scorecard matrices on: (1) problem definition, (2) data and technology readiness, and (3) organizational readiness. Each scorecard helps organizations identify where they fall on a spectrum of four categories ranging from leading to lagging in terms of data readiness. Scorecard categories include: how data is stored; what is collected; privacy and documentation practices; personnel; data use policy; and buy-in from staff, data collectors, leadership, intervener, and funder. (See Appendix A, “The University of Chicago’s Data Maturity Framework”)

- See “Data Maturity Framework” (Center for Data Science and Public Policy) http://dsapp.uchicago.edu/resources/datamaturity/.
- See “Data Maturity Framework Questionnaire” (Center for Data Science and Public Policy) http://dsapp.uchicago.edu/wp-content/uploads/2016/04/Data_Maturity_Framework_4.28.16.pdf.
- See “Introducing Analytics: A Guide for Departments” (City of New Orleans) https://datadriven.nola.gov/datadriven/media/Assets/Docs/NOLAlytics-department-resource-presentation.pdf.
- See “Analytics Excellence Roadmap: A Four-Stage Maturity Model for Data-Driven Government” (Ash Center) http://datasmart.ash.harvard.edu/news/article/analytics-excellence-roadmap-866.
- See “How Data-Driven is Your City?” (Ash Center) https://datasmart.ash.harvard.edu/news/article/how-data-driven-your-city.
3) Scope the Project
Once a department’s data readiness is assessed, it is time to scope the project. There is no one “right” approach to project scoping: in New Orleans, the city’s performance and data expertise team developed a project criteria framework to help scope projects; in the City of Chicago DoIT prioritizes prospective projects by using a framework of research question evaluation criteria. DSaPP’s Data Science Project Scoping Guide has been a particularly successful model for approaching project development and was featured as a pre-conference workshop at the Ash Center’s Inaugural Summit on Data-Smart Government in November, 2017.DSaPP’s Data Science Project Scoping Guide was developed for prospective “Data for Social Good” fellowship projects (a program that supports aspiring data scientists by connecting them with real-world problems) in order to facilitate a continuous pool of well-developed projects that could be successfully deployed within DSaPP’s program cycle. Because each project needs to be scoped thoroughly enough for a data-use agreement, the project scoping steps help expedite project development by providing a concise framework for prospective projects. This project scoping approach helps managers focus on understanding what data is available, who the key stakeholders are for providing and using that data, and how that data is being considered to provide insights into a city governance problem. DSaPP’s four steps for project scoping are:
- Goals – Define the goal(s) of the project.
- Actions – What actions/interventions do you have that this project will inform?
- Data – What data do you have access to internally? What data do you need? What can you augment from external and/or public sources?
- Analysis – What analysis needs to be done? Does it involve description, detection, prediction, or behavior change? How will the analysis be validated?
Chicago uses the Research Question Evaluation Criteria for scoping its predictive analytics projects and determining which projects are best suited for development. Similar to DSaPP’s project scoping steps, Chicago’s evaluation criteria guides project managers through key issues to better situate a prospective analytics project for success. (See Appendix C, “City of Chicago’s Research Question Evaluation Criteria”)

4) Pilot the Project
Piloting an analytics project is “the stuff of innovation.” This is where the trial and error of testing a new project happens. No matter how well prepared an analytics team is, sometimes—whether the problem lies in a key variable, an assumption built into the algorithm, or the project’s general approach—the pilot just does not perform as expected. While information on how best to pilot a municipal analytics project is limited, the frameworks, criteria, and guidelines developed by Civic Analytics Network cities and partners can serve as helpful resources and provide useful examples of how to approach and, ultimately, scale an analytics project.Piloting an analytics project, like any effort to innovate in the public sector, is somewhat at odds with the bureaucratic preference for consistency and risk avoidance, but it is a critical phase that can yield important insights for improving performance when it is time for implementation on a larger scale. Moreover, starting with small-scale pilots can help limit risk and demonstrate clear results.
In an assessment of its pilot practices, the UK Government notes that “Once embarked upon, a pilot must be allowed to run its course. Notwithstanding the familiar pressures of government timetables, the full benefits of a policy pilot will not be realized if the policy is rolled out before the results of the pilot have been absorbed and acted upon.” The report goes on to argue that “pilots should be regarded less as ad hoc evaluations than as early stages in a continuing process of accumulating policy-relevant evidence.”[1]
Piloting also allows for much needed course corrections to help better transition efforts in project-scoping to implementation; adjusting project parameters during the pilot phase can increase the likelihood of success at implementation and beyond.
- See “From Algorithm to Action: How Chicago Operationalized its Predictive Analytics for Food Inspections” (Ash Center) https://datasmart.ash.harvard.edu/news/article/from-algorithm-to-action-759.
- See “Overcoming Barriers to Adoption for Innovations in Policy: Reflections from the Innovation Toolkit” (Innovations: Technology, Governance, and Globalization) https://www.mitpressjournals.org/doi/pdf/10.1162/inov_a_00257.
- See “Piloting the London Office of Data Analytics” (Nesta) https://londondatastore-upload.s3.amazonaws.com/LODA%20pilot%20report.pdf.
- See “Smart City Pilot Projects: Exploring the Dimensions and Conditions of Scaling Up” (Journal of Urban Technology) https://www.tandfonline.com/doi/full/10.1080/10630732.2017.1348884?scroll=top&needAccess=true.
- See “Trying it Out: The Role of ‘Pilots’ in Policy-Making” (UK Cabinet, Government Chief Social Researcher’s Office) https://assets.publishing.service.gov.uk/government/uploads/system/uploads/attachment_data/file/498256/Trying_it_out_the_role_of_pilots_in_policy.pdf.
5) Implement and Scale the Model
Research and literature on implementing and scaling analytics projects remain limited, and given the variability of structures, budgets, and objectives for analytics projects, identifying generalizable practices for scaling these projects is challenging. The Ash Center’s Data-Smart City Solutions and the Civic Analytics Network have begun to capture early lessons from city-level analytics projects, and as more data-driven decision-making projects mature and are replicated, insights into how to improve and scale these leading projects will grow.Currently, Civic Analytics Network cities are working to replicate models implemented by other member cities, and while some of those leading examples are highlighted in this report, further research and use cases on those replicated projects are forthcoming.
- See “A Catalog of Civic Data Use Cases: How Can Data and Analytics Be Used to Enhance City Operations?” (Ash Center) https://datasmart.ash.harvard.edu/news/article/how-can-data-and-analytics-be-used-to-enhance-city-operations-723.
Analytics Project Examples: Public Safety, Housing, Public Health, and Transportation
Public Safety
A city’s public safety capabilities encompass a wide variety of departments and agencies working to maintain the security and welfare of residents. Public safety means more than just having a police department; it is a cross-cutting issue in government, comprising a wide range of services that help prevent and manage crime, coordinate emergency preparedness, secure public transportation systems, and beyond. While the typical resident will likely only engage with their city’s public safety resources, such as the police or fire department, during an emergency, preventative practices, improved internal management, and tech-enabled policies implemented within and by the many departments that comprise a city’s public safety capacities can make the difference between life and death during those times of crisis. In the two examples below, data-driven efforts to strategically distribute smoke detectors and bolster early intervention systems that help supervisors in police departments identify officers in need of training or other mediation offer insights into how Civic Analytics Network cities and partners use analytics to support and improve the efforts of first responders across the country.1) Smoke Detectors and Fire Risk
New Orleans’ Analytics Model Supports Smoke Detector Distribution and National ReplicationAccording to the U.S. Fire Administration, three out of five home fire deaths happen in homes without smoke alarms, and the risk of death from fire in a home is cut in half when a home has smoke alarms. The New Orleans Fire Department (NOFD) provides citizens with free smoke alarms to help bring these life-saving devices to homes in need across the city, but NOFD Superintendent Tim McConnell wanted his department to be more proactive in improving the safety of New Orleans. —
NOFD partnered with the Office of Performance and Accountability (OPA), the city’s data team, to use a predictive analytics model to determine which houses in the city are least likely to have a smoke alarm and at the highest risk of fire fatalities. New Orleans did not have historical data on smoke alarm locations, and culling permitting records yielded limited results. The project had a breakthrough when an OPA team member turned to the U.S. Census Bureau’s American Housing Survey, which includes a question about smoke alarms. When OPA coupled this data with American Community Survey data, the team was able to identify key variables to help predict if a home is missing a smoke alarm and is at high risk for a fire-related fatality. The analytics model developed by OPA has helped NOFD identify on a block-by-block level where to target free smoke detector outreach and distribution efforts. From the project’s start in 2014 to 2016, NOFD was able to distribute and install 8,000 smoke detectors.
New Orleans worked with Enigma, a data analytics startup, to develop the methodology. Since the New Orleans model is based primarily on federal data, Enigma was able to partner with the American Red Cross and DataKind to replicate it across the nation.
- See “Predicting Fire Risk: From New Orleans to a Nationwide Tool” (Ash Center) https://datasmart.ash.harvard.edu/news/article/predicting-fire-risk-from-new-orleans-to-a-nationwide-tool-846.
- See “New Orleans Profile” in “Lessons from Leading CDOs” (Ash Center), 32-33 https://datasmart.ash.harvard.edu/sites/default/files/2018-01/leasons_from_leading_cdos.pdf.
2) Police Early Intervention System
New Early Intervention Systems Help Police Departments Better Manage Officer PerformanceThe University of Chicago’s Center for Data Science and Public Policy (DSaPP) has partnered with Civic Analytics Network cities and others to build and deploy the first data-driven early intervention systems (EISs) for police officers. EISs enable departments to provide training, counseling, and other interventions for officers who are identified as likely to have an adverse incident. Adverse incidents include complaints from a citizen or colleague, use of force or other tactics, or accident or injury. Early EISs only tracked a handful of simple indicators, such as the number of times an officer used force, and omitted context. For instance, the officer’s assigned beat was typically not logged in the system, meaning there was no way to see in the EIS if an adverse incident such as use of force occurred within or beyond the officer’s beat.
DSaPP’s system uses analytics to find predictors of adverse incidents in the department’s data by, for example, tracking which officers respond to the highest number of suicide calls. This allows administrators to assess whether additional training or counselling is needed. DSaPP’s EIS model adapts over time and automatically learns from how supervisors interact with, use, or deprioritize the system’s findings. DSaPP has deployed the system in Charlotte-Mecklenburg and Metropolitan Nashville and, as of spring 2018, is working with two Civic Analytics Network cities to build prototypes.
During the development of the Nashville model, the University of Chicago’s DSaPP team determined that of the approximately 1,000 officers in the city’s department, around five percent have an adverse incident each year. Typical or traditional EISs use a threshold-based model to identify officers in need of intervention, and while that system captures 80 percent of the officers who will have an adverse incident, it does so by flagging almost two thirds of police. A system that recommends that a department retrain two thirds of the police force is simply not a useful administrative tool, and while it can reasonably be argued that the more training a department receives the better, given the already limited resources of many police departments offering universal, continuous training is infeasible. So, given those limitations, the University of Chicago worked to create a more sophisticated system that includes more data, context, and other factors to streamline the EIS results helping department administrators be better equipped to manage their personnel.
The new EIS designed by the DSaPP team still flagged 80 percent of the officers who will have an adverse incident, but targeted only 30 percent of the department for intervention. Additionally, the new EIS assigns a risk score based on past performance, providing an additional level of detail to help supervisors gauge whether an officer needs an intervention. The risk score and EIS are decision-support tools; neither tool replaces the supervisor’s role in forming judgments about officer training needs and performance. Data collection on how this new system has helped reduce adverse incidences is ongoing.
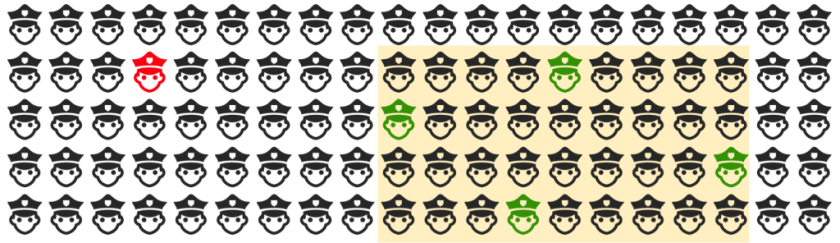
See “Human Lessons Learned Implementing Early Intervention Systems in Charlotte and Nashville” (Center for Data Science and Public Policy) https://dssg.uchicago.edu/2018/03/29/human-lessons-learned-implementing-early-intervention-systems-in-charlotte-and-nashville/.
- See “Police Project Update: Expanding and Implementing the Early Intervention System” (Center for Data Science and Public Policy) https://dssg.uchicago.edu/2017/01/12/police-project-update-expanding-and-implementing-the-early-intervention-system/.
- See “Early Warning/Intervention Systems for Police Departments” (Center for Data Science and Public Policy) http://dsapp.uchicago.edu/2016/02/21/early-warningintervention-systems-for-police-departments/.
- See “Building Data-Driven Early Intervention Systems for Police Officers” (Center for Data Science and Public Policy) http://dsapp.uchicago.edu/projects/public-safety/police-eis/.
- See “UChicago’s Rayid Ghani on Police Incidents, Data Science and Local Government” (YouTube: The University of Chicago) https://www.youtube.com/watch?v=_p9Cz8MJ0Mw.
- See “Identifying Police Officers at Risk of Adverse Events” (KDD) http://www.kdd.org/kdd2016/papers/files/adf0832-cartonAemb.pdf.
Housing
Cities face an increasing need for housing as more and more of the U.S. population moves into urban areas. Providing safe, secure, affordable, and equitably available housing is key to the future stability and success of cities. To this end, Civic Analytics Network cities are using data-driven insights to improve housing conditions in their communities with projects focused on improving housing inspection performance, minimizing overlooked health and safety risks, and remediating blight outbreaks.4) Housing Inspections
San José Uses Analytics to Help Housing Inspectors Keep Residents SafeSan José’s Multiple Housing team in the city’s Code Enforcement Office is tasked with monitoring all properties with three or more units, which totals more than 4,500 properties in the city. It is impossible for the Multiple Housing team to inspect every property each year, so the city partnered with the DSaPP through the Civic Analytics Network to help the inspectors identify properties that are at the highest risk for violations. DSaPP developed a predictive model to provide a view of property violations over time, drawing upon construction permit records and other indicators to develop a model that identifies possible higher-risk properties in the community. The model was developed in close collaboration with the inspectors in San José; DSaPP worked with inspectors, interviewed them, and joined them on inspections to observe how they prioritize and gather information during a site visit. The analytics model DSaPP developed prioritizes violations that pose greater health and safety risks, such as exposed wiring or fire hazards. DSaPP identified four factors that were particularly predictive of higher-risk conditions: (1) amount of time since last inspection; (2) previous health and safety violations; (3) lack of recent building permits; and (4) violation rates of nearby properties. Field tests for this model concluded in late 2017.
- See “Data-Driven Inspections for Safer Housing in San José, California” (Data Science for Social Good) https://dssg.uchicago.edu/2017/07/14/data-driven-inspections-for-safer-housing-in-san-jose-california/.
4) Landlord Discrimination
Analytics Model Focuses on Eliminating Income Discrimination for Prospective Tenants in New York CityIn New York City, Title 8 of the Administrative Code of the City of New York prohibits discrimination based on a number of factors, including race, gender, and religion. The law also includes provisions to protect individuals who receive public assistance from being discriminated against based on their source of income. Despite this, however, income-based discrimination is among the top housing-related complaints in the city.
To curb discrimination against prospective tenants, including tenant harassment, source-of-income discrimination, and illegal conversions of apartments, the Commission on Human Rights partnered with the New York City Mayor’s Office of Data Analytics (MODA) to build a model to help prioritize investigative resources. MODA wanted to identify areas in which landlords were most likely to turn away prospective tenants with housing vouchers—not only to prosecute proven violators, but also to send a clear message that violations of this nature would not go unnoticed and to discourage landlords across the city from discriminating in the first place.
With this in mind, MODA set out to identify the landlords engaging in discriminatory behavior focusing particularly on identifying and punishing larger property management firms. By focusing on identifying the largest landlords making these violations, the city hoped that as a consequence smaller potential offenders would remediate their behavior. MODA’s model has helped the city better identify illegal housing practices, and while the Commission investigates all allegations of income discrimination, MODA’s efforts have helped the Commission better manage their inquiries and, ultimately, improved the city’s ability to curb future incidents of discrimination.
- See “New York City Profile” in “Lessons from Leading CDOs” (Ash Center) Pgs. 34-35 https://datasmart.ash.harvard.edu/sites/default/files/2018-01/leasons_from_leading_cdos.pdf.
- See “The NYC Commission on Human Rights partnered with MODA to assist in identifying sites of potential income discrimination” (MODA NYC GitHub) https://moda-nyc.github.io/Project-Library/projects/source-of-income-discrimination/.
- See “How New York is Protecting Affordable Apartments with Analytics” (Ash Center) https://datasmart.ash.harvard.edu/news/article/how-new-york-protecting-affordable-apartments-analytics.
5) Blight Remediation
New Orleans Uses Data-Driven Insights to Reduce BlightAfter Hurricane Katrina in 2005, rebuilding New Orleans was a daunting, arduous endeavor, and many areas that were damaged by the wind and flooding were left untouched in that state for years. As a result, rampant blight took hold in and around those damaged properties across the city. When Mayor Mitch Landrieu took office in 2010, blight remediation was a top priority, and the Office of Performance and Accountability (OPA), the analytics hub of New Orleans’ city government, was tasked with developing analytics and performance management tools to help tackle this dangerous housing issue.
OPA developed BlightSTAT as a performance management tool to improve services and results through the use of data. OPA also created a Blight Scorecard to address a backlog of more than 1,500 properties awaiting a decision by the Department of Code Enforcement. The Blight Scorecard allows mid-level supervisors to score a property on several dimensions and then receive a weighted recommendation between 0 and 100, 0 meaning the property should be demolished and 100 meaning it should be sold. Those mid-level supervisors and their superiors can use the tool to evaluate properties, vastly increasing the speed and consistency of the process at various levels in the department. Like Chicago Police’s EIS, the scorecard is a decision-support tool and does not replace human judgment—it provides a recommendation, and the director reviews the available information and decides whether to approve each demolition.
This new tool has improved the workflow of the city’s Code Enforcement Department by directing negative judgments to supervisors for review prior to escalating them to the attention of the director. Moreover, the new process adds efficiency by removing all paper components. Use of this tool effectively eliminated the backlog of blighted properties in the city. Other cities are also tackling blight remediation with analytics; for example, Cincinnati partnered with the University of Chicago’s DSaPP via the Civic Analytics Network to develop an early detection model.
- See “New Orleans Brings Data-Driven Tools to Blight Remediation” (Ash Center) https://datasmart.ash.harvard.edu/news/article/new-orleans-brings-data-driven-tools-to-blight-remediation-915.
- See “Code Enforcement Abatement Tool” (City of New Orleans) https://www.nola.gov/performance-and-accountability/reports/nolalytics-reports/nolalytics-blight-abatement-tool-brief/.
- See “Blight Prevention: Building Strong and Healthy Neighborhoods in Cincinnati” (Center for Data Science and Public Policy) https://dssg.uchicago.edu/2015/08/20/blight-prevention-building-strong-and-healthy-neighborhoods-in-cincinnati/.
- See “Proactive Blight Reduction and Neighborhood Revitalization: City of Cincinnati” (Data Science for Social Good) https://dssg.uchicago.edu/project/proactive-blight-reduction-and-neighborhood-revitalization/.
- See “Early detection of properties at risk of blight using spatiotemporal data” (Data Science for Social Good) https://dssg.uchicago.edu/wp-content/uploads/2016/10/34_blancas.pdf.
Public Health
From the risk of foodborne illness to viral outbreaks, mitigating public health concerns is a matter of good, forward-looking governance. The global health risk posed by mosquito-borne viruses like Zika and West Nile is immense, and as the impact of climate change becomes more overt the risk of such viral outbreaks will only grow. Forecasted population and environmental changes pose unprecedented challenges for local governments, but analytics can provide critical insights to help cities prepare for and curb the impact of future outbreaks.Monitoring food quality and safety in a city are similarly challenging tasks, due to the complexity of regulations for food preparation, storage, service, and so on. Cities across the Civic Analytics Network have developed analytics models to help city officials deploy their limited resources more effectively to monitor and manage restaurant inspections and pest control. By forecasting areas at risk for mosquito-borne disease, rodent infestation, and poor food quality, cities are able to better manage their limited resources and provide their communities with a safer, healthier quality of life.
6) West Nile VIrus
Chicago Uses Analytics to Prepare for Forecasted Spike in West Nile VirusBy the end of this century, Chicago’s climate is projected to become so warm that it will mirror the conditions seen in present-day Alabama. As warmer climates shift northward and expand mosquito-friendly environmental conditions, the risk of mosquito-borne viruses will increase considerably. The forecasted spike in mosquito population in the Chicago area helped prompt Chicago’s Department of Innovation and Technology (DoIT) to partner with the Chicago Department of Public Health (CDPH) to use data to find new solutions for this projected public health issue. The city built a predictive model to determine one week in advance whether or not a particular area will have West Nile virus-carrying mosquitoes. With such a model, Chicago can direct mosquito-spraying efforts towards areas that pose the greatest risk, mitigating the potential of a viral outbreak.
The concept for Chicago’s West Nile virus analytics project came via Kaggle, an online platform for analytics and predictive modeling competitions. In early 2015, CDPH, in partnership with DoIT, launched a Kaggle competition of its own by posting some West Nile virus visualizations and starter code in R (a program language and software for running statistical analyses) and Python (a high-level programming language for general computer program and analyses) on Kaggle Scripts. In 2017, following a successful pilot program, Chicago operationalized its West Nile virus analytics model. Chicago’s efforts to combat West Nile virus can potentially be replicated to help cities manage public health risks associated with other mosquito-borne illnesses, including the Zika virus. Chicago’s model along with instructions for running it are available on the city’s GitHub page.
- See “Predictive Analytics Guides West Nile Virus Control Efforts in Chicago” (Ash Center) https://datasmart.ash.harvard.edu/news/article/predictive-analytics-guides-west-nile-virus-control-efforts-in-chicago-1152.
7) Zika VIrus
Zika Outbreak Triggers Coordinated Analytics Response from Cities across the U.S.The Zika virus burst onto the international stage in 2016 as a global health emergency. While Zika is not a new virus, the unprecedented outbreak in 2016 brought this disease to the fore of public health debates around the globe. While travel-related Zika cases in the United States remain limited, local health officials and urban policymakers alike must grapple with the risk that Zika-infected mosquitoes pose to their communities. Zika is transmitted by the Aedes aegypti mosquito, which thrives in warm, wet climates. So, like West Nile virus, the risk of Zika in cities across the country will likely only grow in the years to come as climates become warmer around the globe.
Unlike Chicago’s forecasted West Nile virus risk, New Orleans is already home to abundant Aedes aegypti populations. New Orleans, like most cities, has well-established mosquito monitoring systems in place, but following the 2016 Zika outbreak additional methodologies and resources for controlling, monitoring, and predicting population spikes were needed. For example, New Orleans is using ArcGIS (a geographic information system, GIS, software system) to create risk maps based on analytics to help identify baseline larval habitats and to streamline sampling after the city intervenes with a population.
National analytics strategies for addressing the risk of the Zika virus are still being structured, but a key first step for successfully deploying analytics in this area is to identify risk factors that could potentially exacerbate climate-based and warm season outbreaks. For example, in his Data-Smart City Solutions article, Jonathan Jay argues that analytics that correlate mosquito populations with vacant property areas can help cities pinpoint areas at high risk for an outbreak. Integrating or replicating existing analytics models is a likely next step. For instance, New Orleans is already using analytics to tackle blight, and linking this housing-specific effort to public health data could provide invaluable insights into mosquito-borne disease outbreaks and how to curb them.
- See “How U.S. Cities Can Target Zika Risk” (Ash Center) http://datasmart.ash.harvard.edu/news/article/how-u.s.-cities-can-target-zika-risk-960.
- See “Condensed Zika Virus Plan” (City of New Orleans) https://www.nola.gov/getattachment/Health/Emergency-Preparedness/Zika/Condensed-Zika-Plan-8-3-16.pdf/.
8) Restaurant Inspections
Chicago Optimizes Restaurant Inspections with AnalyticsChicago has more than 7,300 restaurants within city limits, plus thousands of grocery stores and other food vendors. Despite the fact that the number of food establishments in Chicago totals more than 15,000, however, the city only employs three dozen inspectors. With no additional resources available for restaurant inspectors, the city needed to work smarter to improve its inspection performance. Following media reports highlighting poor food quality and inspection performance in restaurants, city officials focused on finding new solutions to help improve inspection processes. Chicago’s Department of Innovation and Technology (DoIT) worked with the Chicago Department of Public Health (CDPH) to develop an analytics model that would help forecast restaurants’ risk of failing inspection. The model, developed in partnership with AllState’s pro bono team, enabled the city to identify critical violations an average of seven days earlier than the previous process for ordering the inspections. In addition, Chicago’s Data Portal (the city’s open data hub) houses a Food Inspections tracker to provide the community with transparent, real-time information on the food inspection results for city restaurants.
- See “Delivering Faster Results with Food Inspection Forecasting” (Ash Center) https://datasmart.ash.harvard.edu/news/article/delivering-faster-results-with-food-inspection-forecasting-631.
- See “Food Inspections” (Chicago Data Portal) https://data.cityofchicago.org/Health-Human-Services/Food-Inspections/4ijn-s7e5/data.
- See “Lessons from Leading CDOs” (Ash Center) Pg. 29, https://datasmart.ash.harvard.edu/sites/default/files/2018-01/leasons_from_leading_cdos.pdf.
9) Pest Control
Chicago’s DoIT Team Fills Information Gap with 311 Data to Curb Rodent InfestationsThe City of Chicago’s Department of Innovation and Technology (DoIT), in partnership with Carnegie Mellon University’s Event and Pattern Detection Laboratory, developed predictive analytics tools to support efforts to combat rodent infestations in Chicago and to help the city develop more effective rodent baiting programs. Because the city had limited formal information documenting rodent populations, geography, and other factors critical to helping city officials tackle infestations, DoIT utilized 311 data in its analytics model to fill the information gap.
311 call data is the foundation of the city’s rodent infestation analytics model and helps the city determine where rodent infestations could exist and predict where those populations could spike. Geospatial representations of this information support the Department of Streets and Sanitation’s rodent baiting program. Sean Thornton, a program advisor for the Civic Analytics Network based in Chicago, notes, “In July 2013, Mayor Rahm Emanuel announced that as a result of the City’s increase in preventive rodent baiting efforts in 2012, resident requests for rodent control services have dropped 15% in 2013.”[2]
- See “Using Predictive Analytics to Combat Rodents in Chicago” (Ash Center) https://datasmart.ash.harvard.edu/news/article/using-predictive-analytics-to-combat-rodents-in-chicago-271.
- See “Chicago Profile” in “Lessons from Leading CDOs” (Ash Center) Pgs. 29-30, https://datasmart.ash.harvard.edu/sites/default/files/2018-01/leasons_from_leading_cdos.pdf.
Following a surge in 311 calls related to rodent sightings in 2016, the District of Columbia’s city government decided to use analytics to help combat the city’s rat problem. The Lab @ DC, situated in the Office of the City Administrator, and the Office of the Chief Technology Officer (OCTO) work together on various city priorities and projects to develop data-driven solutions to help city government better serve the District. As part of this broader effort, the Lab @ DC and OCTO data scientists partnered with the rodent control team at the Department of Public Health to develop an analytics model to identify environmental variables that help predict where a rat infestation is likely to develop. To build an analytics model, the city is using 311 data, and, similar to rodent infestation management efforts in Chicago, D.C. is working to identify leading indicators within 311 data to support that model. The city’s efforts to develop an analytics model to support rat abatement are ongoing.
- See “To squash rat outbreak, D.C. turns to technology” (StateScoop) http://statescoop.com/to-squelch-rat-outbreak-d-c-turns-to-technology.
- See “Rat problems? In D.C., data could help” (GCN) https://gcn.com/Articles/2017/08/01/Lab-DC-Leonard.aspx.
- “Mayor Bowser Highlights Citywide Efforts to Reduce Rodents” (DC.Gov) https://mayor.dc.gov/release/mayor-bowser-highlights-citywide-efforts-reduce-rodents
Transportation
Rising urban populations and new technologies such as automated vehicles are pushing cities to rethink their transportation systems and regulations to ensure driver and pedestrian safety. New mobile applications, technologies, and modes of transit are revolutionizing how residents traverse their home cities, and it is increasingly apparent that existing city resources and regulations are insufficient to deal with these new practices. Outdated public transit and transportation infrastructure is a common issue in cities across the U.S., and while a cross-cutting strategy with immense funding support is required to address this issue, data analytics can offer a cost-effective way forward for cities. Transportation and data analytics go hand and hand, and the wealth of data available in transportation is a critical and in many cases low-cost resource that can help city officials assess and improve transportation systems. Cities need to be equipped with the right personnel and tools to use analytics to understand and help regulate existing and new transportation modes and patterns.In parallel to city efforts to understand the implications of new technologies and services in transportation, a global movement called Vision Zero is focused on eliminating traffic fatalities. As part of this movement, which originated in Sweden in 1997, cities across the Civic Analytics Network, from Boston to Los Angeles, are developing analytics models and visualization platforms to make progress toward this goal. How cities will grapple with the implications and challenges of automated vehicles along with the complexity of multi-modal, shared roads remains to be seen, but municipal analytics models that are being developed can help prepare cities for the changes brought by these and other new technologies.
10) Traffic Collisions: Vision Zero
New York City’s Analytics Team Aims for Zero Traffic Fatalities with Exposure MapAs part of the Vision Zero movement, New York City began developing an analytics model in 2015 with DataKind to enable city officials to assess whether a new transportation project is high risk (i.e., likely to result in injury or unsafe traffic circumstances) and to estimate the likelihood of various outcomes. For example, if the Department of Transportation (DOT) is developing a new intersection or traffic lane design, the analytics model assesses the risk of injury in that intersection or lane design, allowing project managers to adjust the design and recalculate to get the safest results while still accounting for need to improve traffic patterns and minimize delays.
While limited data rendered this initial project design unsuccessful, the model’s ability to calculate the number of cars on a road produced a useful exposure model that is being used by the city’s DOT to more accurately determine the effectiveness of different street designs. The exposure map allows the DOT to forecast the number of cars on the road to help better manage traffic patterns and improve road safety for pedestrians and drivers alike.
- See “Can Better Data Make Zero Traffic Deaths a Reality?” (Ash Center) https://datasmart.ash.harvard.edu/news/article/can-better-data-make-zero-traffic-deaths-a-reality-1138.