In this month’s installment of the Innovation of the Month series, we explore the work of Case Western Reserve University (CWRU) on their α-satellite project, designed to provide real-time risk assessment of locations across the country to aid social distancing and reopening strategies amid COVID-19. MetroLab’s Ben Levine spoke with Professor Fanny Ye and Professor Ken Loparo about the approach and development of their project.
Levine: Can you describe the origin and objective of the α-satellite project and who has been involved in it?
Fanny Ye: In late February, as the coronavirus was continuing to spread globally and the first death was reported in the U.S., we realized that there was a real potential for a novel coronavirus pandemic like what occurred in the 1918 flu pandemic. A growing number of areas reporting community transmission would represent a significant turn for the worse in the battle against the virus. We sought to answer a core question as we witnessed the development: As computer scientists, how can we advance capabilities of artificial intelligence (AI) and leverage the large-scale and real-time data generated from heterogeneous sources for the development of computational tools to assist with community mitigation?
In early March, I initialized the discussion with Professor Ken Loparo regarding the ideas of real-time COVID-19 risk assessment and we began to lead the team for the design and development of a project called α-satellite (alpha satellite), aimed at providing real-time COVID-19 risk assessment in a hierarchical manner to provide individuals and communities actionable strategies to slow the spread of viral infections. “Hierarchical manner” in this context means that, given a location (either provided by the user or automatically gathered from their device), α-satellite will automatically provide the level of risk, or risk indices, associated with the specific location, the county that location is in and the state as a whole to enable people to select appropriate actions for protection while minimizing disruptions to daily life to the extent possible. The team members include five Ph.D. students and two master’s students in my lab.

Hierarchical risk assessment of a user's current location, the corresponding county, and the state as a whole.
Levine: There are so many challenges presented by the COVID-19 pandemic. Which of them is your project addressing?
Ye: According to the Centers for Disease Control and Prevention (CDC), before a vaccine or drug becomes widely available, community mitigation, which is a set of actions that persons and communities can take to help slow the spread of respiratory viral infections, is the most readily available intervention to help slow transmission of the virus in communities. Among many challenges being presented by the COVID-19 pandemic, the objective of α-Satellite is to provide dynamic and real-time COVID-19 risk assessment to facilitate community mitigation and slow the spread of viral infections.
Ken Loparo: More specifically, social distancing is an effective way to mitigate the spread of the novel coronavirus only if it achieves compliance. In other words, it only works when the population follows the suggested guidelines and restrictions that are being suggested or required. We realized that during the period of social distancing, people were still going to need to visit certain establishments such as grocery stores, pharmacies, etc., to meet their essential needs. In considering approaches for addressing this problem, we found an AI-driven system leveraging large-scale and real-time data collected from multiple sources would provide real-time COVID-19 risk indices associated with the areas that users query at three levels: the user’s specific location, county and state. The information, in combination with other community, county, city, state and federal guidelines, would help individuals practice social distancing while still meeting their needs for obtaining essential items for a variety of different establishments.
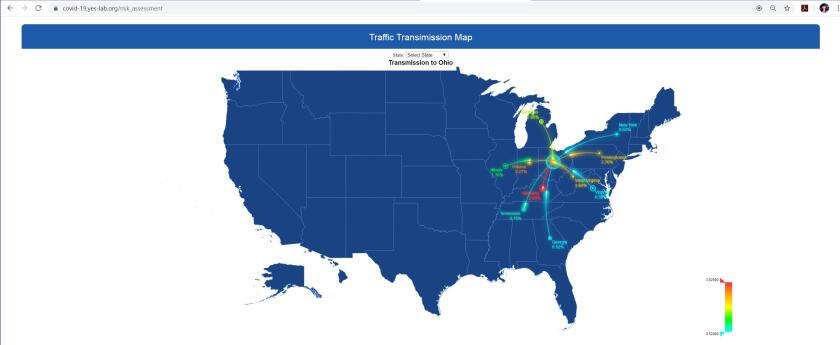
Traffic Transmission Map of geographic risk surrounding Ohio.
Levine: What kinds of data sources are you using? How are these different from other COVID visualization projects?
Ye: In the first prototype of α-satellite, the decision was made to use publicly available data sources including 1) disease-related data: up-to-date county-based coronavirus-related data including the numbers of confirmed cases, new cases, deaths and the fatality rate from official public health organizations such as the World Health Organization, CDC, state and county government websites, and digital media; 2) demographic data: the data obtained from the United States Census Bureau including basic population, business and geography statistics for all states and counties, and for cities and towns with more than 5,000 people; 3) mobility data: a mobility measure that estimates how busy a given area is in terms of traffic density; and 4) user-generated data from social media (e.g., Reddit) to learn public perceptions toward COVID-19. The basic idea behind using these as the initial data source is that, for example, population density would provide insight into the baseline population in the area/location you are contemplating visiting; the traffic density data would provide additional information related to human activity in the area, and the public perception data would provide an assessment of the general COVID-19 awareness of people in the area you are investigating. For instance, given similar severity of COVID-19 cases, if the population and traffic density in an area are high and public perception is low (meaning either people are unaware about COVID-19 or do not believe that COVID-19 is serious), the relative risk of this area would be higher than other areas with lower population and traffic densities and higher public perception.
Different from other COVID-19 visualization projects, a majority of which are only providing static mapping, α-satellite is the first attempt to provide dynamic and real-time COVID-19 risk assessment associated with any given area in a hierarchical manner. Leveraging the large-scale and real-time data generated from heterogeneous sources, the system utilizes advanced, AI-driven techniques to provide real-time COVID-19 risk estimation for any given area in the U.S.
Levine: Who are you working with in the Cleveland region to put this project to use?
Loparo: The first prototype of α-satellite was developed and deployed on April 1; we launched our system to the public for beta testing on April 20. Our plans are to work with the city of Cleveland and other cities and communities in the coming weeks and months. As the plans and strategies for reopening are ongoing, an AI-driven tool like α-satellite should provide a good source of both data and information to assist individuals with decision-making as they contemplate how to return to the “new normal.”
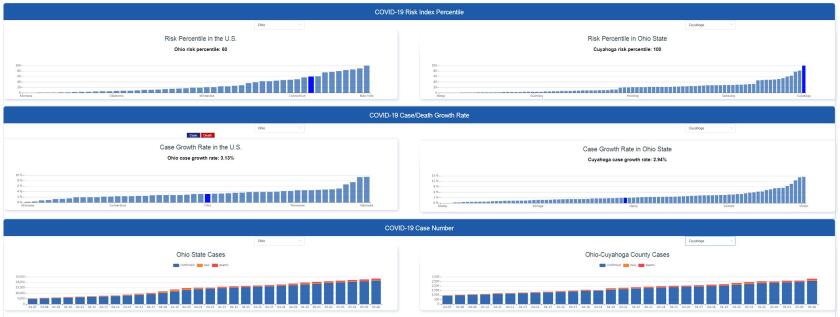
COVID-19 Risk index relative to other locations throughout the U.S.
Levine: What challenges did you face in developing this tool?
Ye: There have been many challenges we have faced during the development and deployment of α-satellite, including assuring the stability and scalability of the system to support a large volume of user visits, large-scale and up-to-date data collection and preprocessing, algorithm improvement, new functionality design, and server and database deployment. The workload is more than we initially imagined, and the team has been working extremely hard.
Levine: What results are you finding? Are any particularly surprising?
Ye: We are getting a lot of good feedback from the media and users on the ease of use of the tool as well as the utility of the relative risk estimation. After we launched our system to the public for beta testing on April 20, it had attracted 42,546 users in the first week. This indicates the public’s high demand for effective computational tools to assist with actionable strategies for protection while minimizing disruptions to daily life.
Loparo: A common question from users is how to interpret the results in terms of comparisons between risk indices for different locations. The important point here is the concept of relative risk; that is, the numeric risk index of a particular location is only meaningful when compared to the numeric risk index at another location, generally in the same city or county. Depending on the availability and granularity of the data, various components in the numeric risk index may be the same or very similar, so that the relative differences in the indices may not be as significant as expected. When comparing the risk indices of specific areas in different counties/states, in addition to the numeric values for those areas, users can also compare the state and county values associated with the two areas. For example, at 6:25 p.m. on May 4, given a grocery store with medium traffic in Cleveland with the risk index of 0.723, the information of its related county and state can also be considered; i.e., Cuyahoga County has a risk percentile of 100 in the state indicating the highest risk among all the counties in Ohio, while the state of Ohio has a risk percentile of 50 in the country, indicating a medium-level risk among all states in the U.S. As we include more location-specific data, the computations will provide additional comparative detail. However, it is important to recognize that with the possible increase in discriminatory detail between locations comes the concomitant risks to privacy, security and accuracy. It is important here that trustworthy data is used in the computations to avoid any issues that can arise from malicious intent.

ANNIE ONEILL PHOTOGRAPHY
CWRU α-satellite team in Dr. Ye's lab.
Levine: Where do you see this project going from here? What are your next steps?
Loparo: With the discussions and development of when and how various cities and states will approach reopening, α-satellite provides unique opportunities for individuals to decide how they plan to move forward, based on their personal preferences, in returning to a more normal lifestyle. It is evident that there is a diversity of opinions on the appropriate plan for reopening, not only between state and federal leadership, but also among individuals on how they will react to and adopt reopening strategies in their community. α-satellite was originally envisioned to provide individuals guidance on how to facilitate community mitigation while meeting essential needs as COVID-19 was spreading and ramping up in communities. As we now move to relax stay-at-home restrictions while still respecting social distancing requirements, α-satellite will provide a unique opportunity in assisting individuals in making personal decisions with regard to how they will transition to and resume to “normal” activities.
Ye: We just launched α-satellite (V2.0) on May 4 with new features of static board for COVID-19 comparisons and trend analysis for user-customized counties and states. The new features will assist not only individuals, but also organizations in decision-making for the reopening. It’s relevant to note that our team was given a prestigious award from the National Science Foundation (NSF) on May 1 in support of our COVID-19 risk assessment project. The award comes with $84,000 in funding over a one-year period. In our next steps, with this support from the NSF, we will focus on refinements to data sources and algorithms to effectively utilize all available data to improve the accuracy of real-time risk estimation. We also plan to work with city and state governments as well as health-care providers in the future applications of the α-satellite concept as it relates to other important public health-related issues.